The Université Jean Monnet (UJM) team in the frame of Premiere project develops a comprehensive set of video processing and 3D reconstruction tools, including 2D pose estimation, 3D pose, tracking and trajectory estimations, combining advanced computer vision methods with AI tools, such as Deep Neural Networks (DNN), to analyse and model contemporary dance video content. The work also addresses creating 3D models for static elements in audio-visual archives, aiming to integrate these representations into future analysis tools. The ultimate goal is to generalise these methods for broader audio-visual content, such as theatre, and to develop tools that help choreographers and dancers analyse and document dance movements, potentially creating real-time avatars for virtual or extended reality performances.
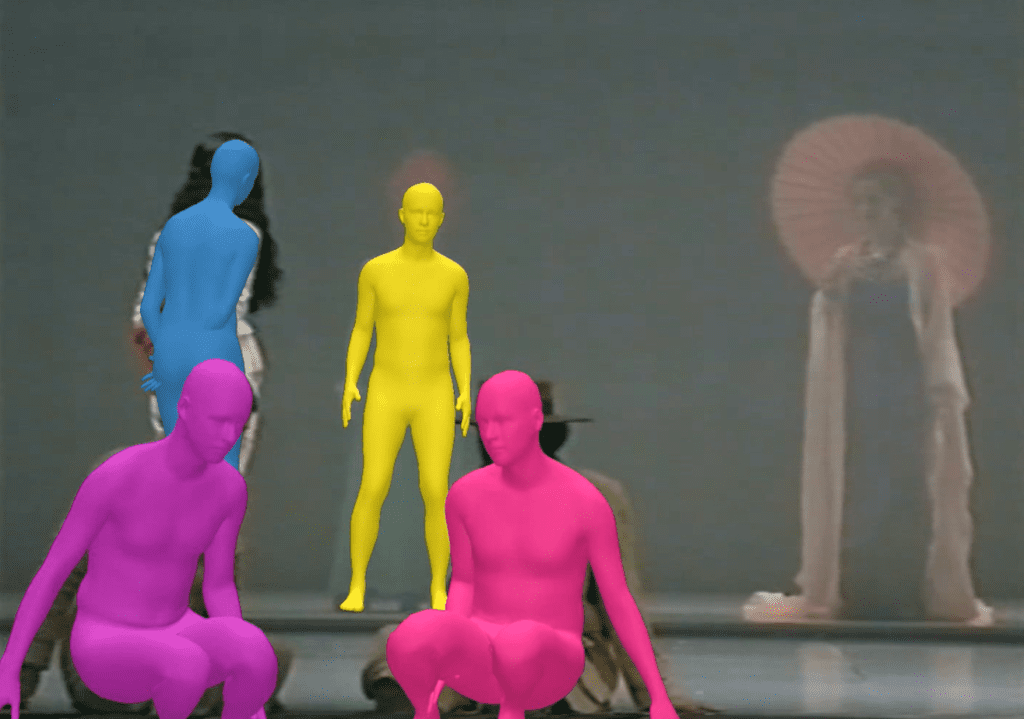
While the efficiency of cutting-edge architectures, algorithms and tools has been demonstrated in the literature, their robustness to challenges such as lighting conditions, occlusions, motion blur, frame rate, image quality, video content, video resolution, costumes elements, etc. has not been yet fully investigated, especially in the context of contemporary dance. The team’s objective was therefore to propose a framework of tools that leverages the limits of existing approaches.
Motion Capture (MoCap) systems are widely used in various fields, such as performing arts, entertainment, sports, healthcare, etc. Traditionally, Motion Capture systems rely on expensive and complex hardware that can only be operated by trained professionals. However, with the advancements in 3D computer vision, it has become possible to use low-cost cameras and software to achieve accurate 3D motion capture.
For a few years there has been a general trend in the domain of Computer Vision to use Machine Learning and Artificial Intelligence tools for this task. Very recently several efficient methods have been published in the literature addressing the challenges related to 3D human body pose estimation, human body tracking and trajectory estimations. Very few of these cutting-edges methods addressed the application field of dance and of performing arts in general.
Among the many objectives that were set for the PREMIERE project, the Laboratoire Hubert Curien at UJM has been in charge of two challenging aspects: 3D scene Analysis and Understanding, as well as 3D pose trajectories estimation in complex scenes.
The 3D scene Analysis and Understanding task involved creating a dataset of live performances representatives of complex dance or theatre events (with multiple persons), using several cameras positioned at different points of views. The annotation (labelling) of these audio-visual contents will enable our team to test, compare and retrain Convolutional Neural Networks (CNN) designed for these tasks.
The 3D pose trajectory estimation in complex scenes involved building a 3D model of moving people in these audio-visual contents from the different available views. Our lab’s team then evaluated the potential of the investigated and implemented methods. The most promising 3D scene analysis and understanding methods – including multi-people pose and motion estimation, segmentation and tracking methods, action parsing methods – were tested, compared and evaluated. Experimental results obtained from single-view methods (and archives) and multi-views methods were compared and analysed.
The third challenge addressed the building of a 3D model for static elements present in audio-visual archives. Our lab’s researchers tested, compared and evaluated the most efficient 3D scene analysis and understanding methods, including object detection methods, segmentation or tracking methods. In the next six months, these 3D representations of static elements will be integrated as additional data for the algorithms used in the analysis, understanding and 3D reconstruction of audio-visual contents.
The fourth challenge currently under investigation aims to define a set of generic methods and tools that could be generalised or transferred to other audio-visual contents or study cases – depending of the complexity of their content – beyond those explored as part of the PREMIERE project. The objective will be to evaluate the efficiency and performance of the methods investigated and implemented, in order to establish a set of rules linked to various factors (events’ content, costume elements, lighting conditions, data quality, etc.) which will help determine the functionalities that could be extended to other events with minimal modifications, those that would require adaptations, or those that would be unusable. In the next steps, our lab’s team will further investigate the potential of individual object pose (such as human body or hands) and methods such as motion estimation, human body pose trajectory estimation, tracking (methods based on 3D keypoint detection, kinematic skeleton, bounding box, semantic segmentation, 3D template model), action parsing, etc.

The focus will extend to finding solutions for handling inter-object occlusions, abrupt motion changes, appearance changes due to varying lighting conditions between viewpoints, low contrast with the background, missing detections resulting from non-rigid deformation of clothing, truncations of persons, or interactions between people.
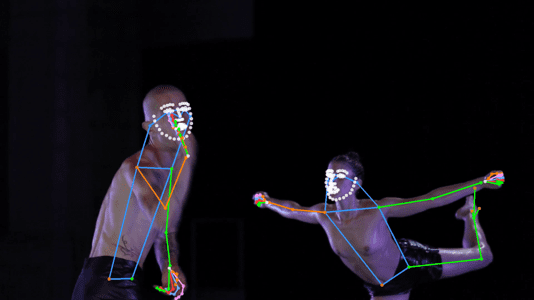
The mid-term objective is to define a set of generic video processing tools that could be generalised/transferred to other categories of audio-visual contents than contemporary dance video contents, such as theatre video contents. The long-term objective is to develop a set of generic video processing tools that could enable choreographers and dancers to understand, describe, interpret and document how dancers move their body, i.e. to automatize the process of dancers movements analysis; – to create in real time avatars of dancers during a performance in virtual reality or extended reality. Finally, the Laban Movement Analysis (LMA), could potentially be added to the 2D video content analysis. Laban’s method*, with other techniques taught in dance schools, enables us to understand, describe, interpret and document how humans move their body.
By Laboratoire Hubert Curien – University Jean Monnet (UJM) team (Philippe Colantoni, Damien Muselet and Alain Trémeau)