In the Laboratoire Hubert Curien at Saint-Etienne the first tests and experimentations done on the Thomas Talawa Prestø video provided by Amsterdam University of the Arts enable to demonstrate that applying 2D human body pose estimation technique, based on deep learning method, on dance scenes we can:
- Learn how many dancers are performing in each sequence of the video;
- Estimate if each body part is visible in each frame of a sequence;
-
Estimate if each body part is visible in each frame of a sequence;
- Estimate the position in the space of the dancer (the best position for data analysis in when the dancer is in front of the camera, his/her face fully visible, in a vertical position without any occlusion between body parts);
- Model each human body shape (or parts of the body) by a skeleton model and a set of reference keypoints (a skeleton model can be used to create a 3D avatar of each dancer).
The team also demonstrated:
- The robustness, the accuracy and the invariance of several UpToDate 2D human body pose estimation technics against specific positions of dancers in the space, changes of lighting conditions, shadows and ground reflections, etc.
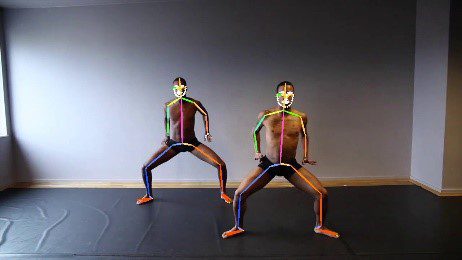
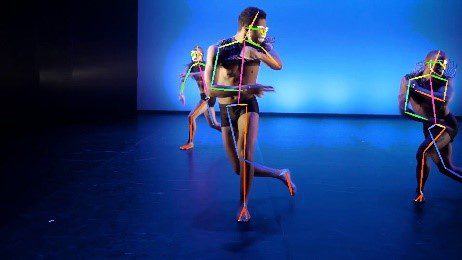
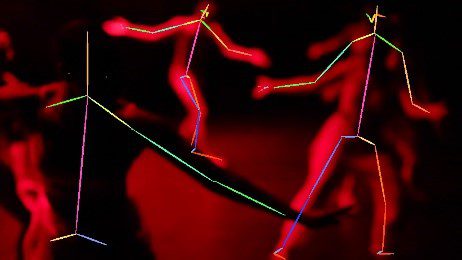
Examples of lighting invariance of the skeleton models tested
- The limits of 2D human body pose estimation from one view camera versus 3D human body pose estimation and 2D human body pose estimation from multiview cameras.
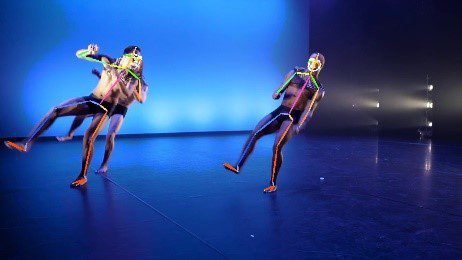
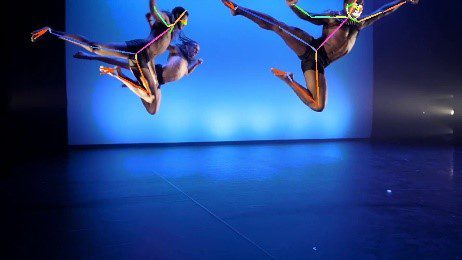
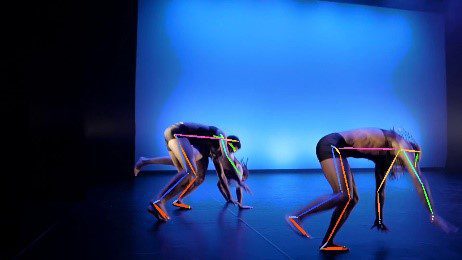
Examples of invariance of 2D human body pose estimation methods to “unconventional” body positions.
Further experiments were made with a multiview setup based on 10 GoPro cameras (synchronised and geometrically calibrated). The main advantage of this kind of motion capture systems is that it is markerless (less constraining for dancers).
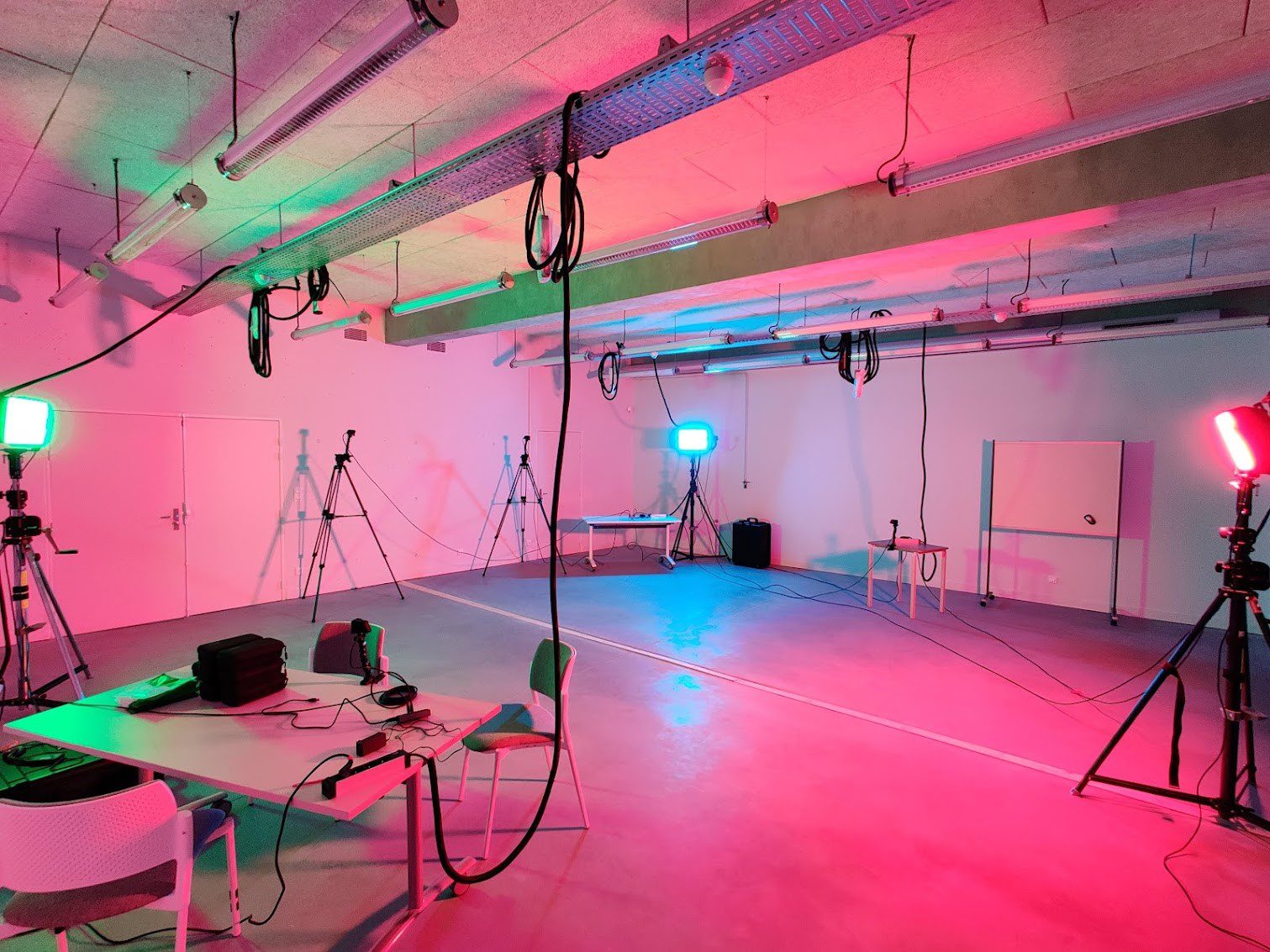
Example of acquisition and lighting setup.