The Université Jean Monnet (UJM) team is working on the creation of a Dance Motion Dataset composed of live performance video recordings. These recordings introduce variations of lightning conditions, pose and occlusion patterns, tackling important challenges in human body pose estimation.
On 21 Sept. 2023, UJM team recorded a set of video sequences at AHK ID-Lab Amsterdam. Two professional dancers, Jeroen Janssen and Rodrigo Ribeiro, performed first individually, next together, in front of a multi-view setup. They were asked to perform first contemporary dance, next specific body poses and intertwining of bodies.
The objective was to create a live performance dataset of video contents (titled “PREMIERE Dance Motion Dataset”) with specific features that are not covered by the AIST++ Dance Motion Dataset (as reported in the Table below).
The AIST++ Dance Motion Dataset contains 1,408 human dance motion sequences on 10 dance genres with hundreds of choreographies, acquired from 9 views. It provides camera intrinsic and extrinsic parameters computed from a 3D calibration process based on keypoints. Motion durations vary from 7.4 sec. to 48.0 sec. In total, this dataset contains 10,108,015 frames of 3D keypoints with corresponding images.
The following figures illustrate the added features.
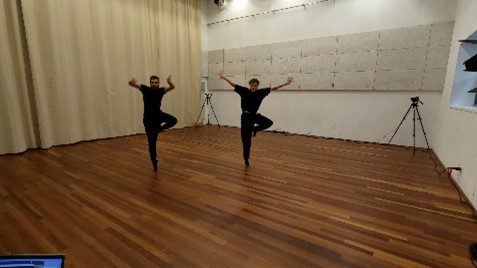
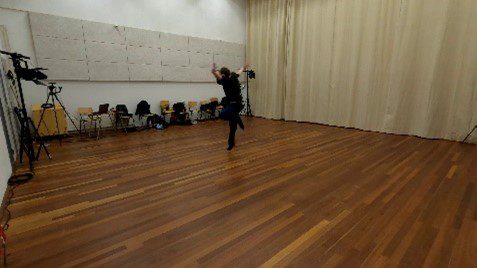
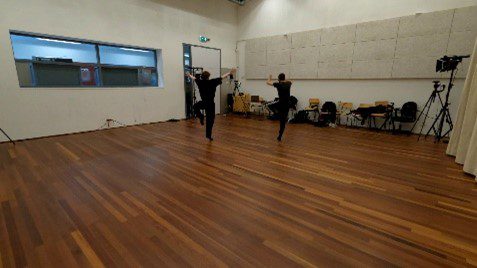
Figure 1: Images of the same video sequence from different viewpoints. (a) from front camera (this view is the best one to estimate the orientation of body shapes and faces emotion), (b) same image from side camera (here we have a strong occlusion between dancers), (c) same image from the back.
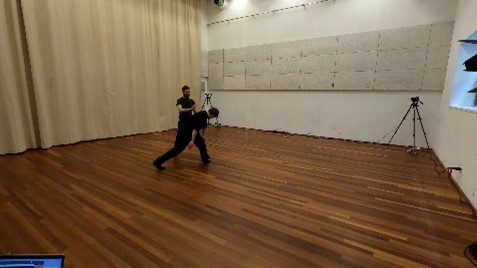
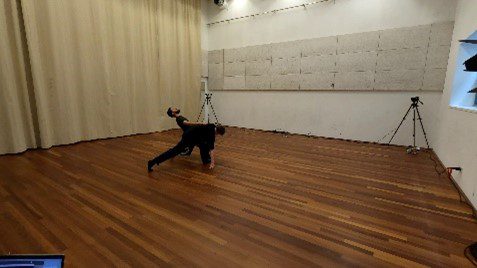
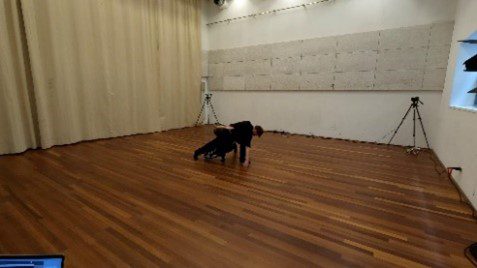
Figure 2: Images from another video sequence. (a) one of the dancers occludes the lower part of the body of the second dancer, (b) complex occlusion pattern, (c) another challenging occlusion pattern. Images from the PREMIERE Dance Motion Dataset.
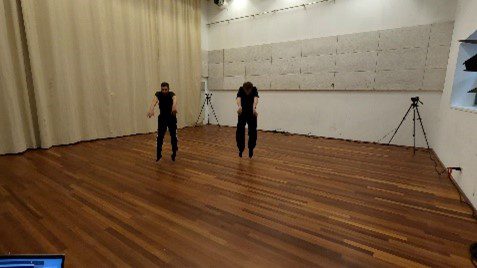
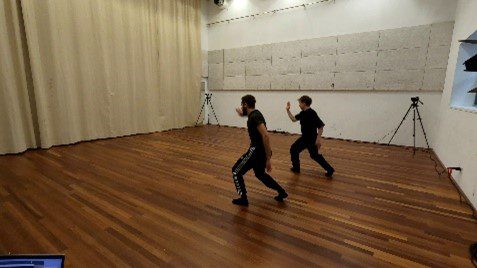
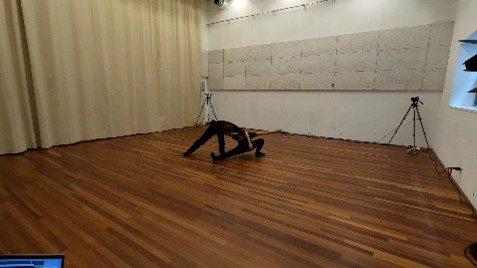
Figure 3: Images from another video sequence. (a) The feet of the dancers are not connected to the ground, (b) motion blur for one of the hands, (c) complex pose of the dancers. Images from the PREMIERE Dance Motion Dataset.
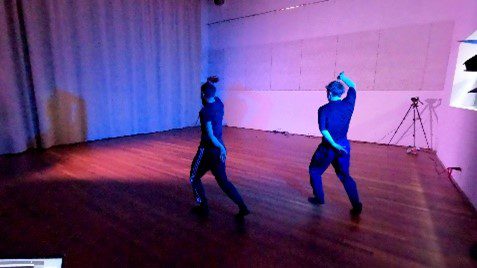
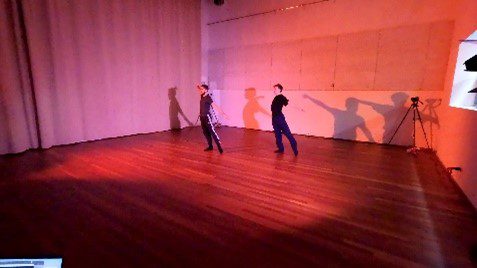
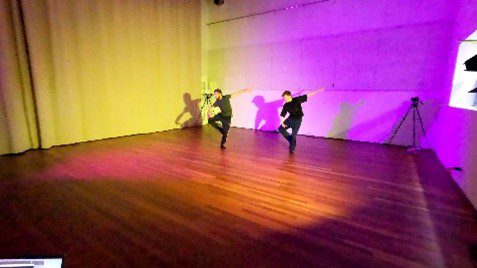
Figure 4: Images from another video sequence taken using with two asynchronous color spots. (a) dancers are in the shadow area, (b) color shifts induced by changes of lighting color over time, (c) complex shading effects induced by changes of lighting color over time. Images from the PREMIERE Dance Motion Dataset.
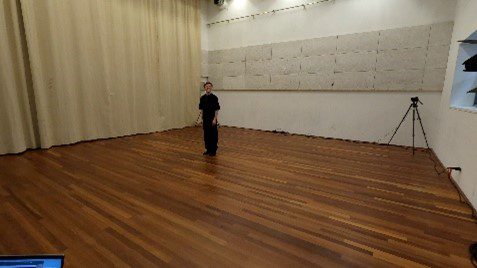
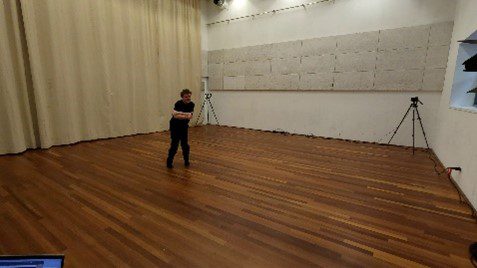
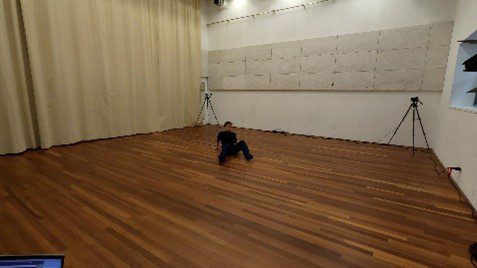
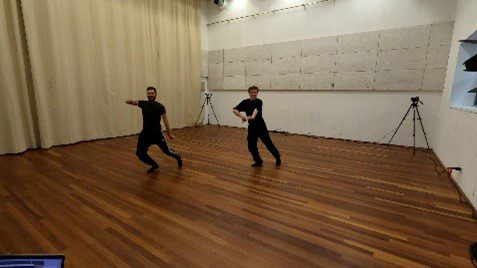
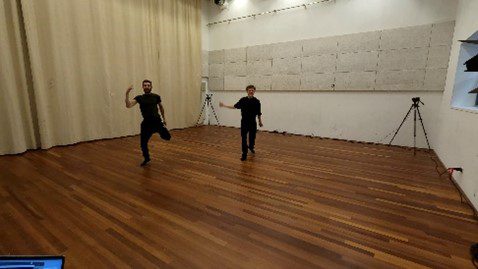
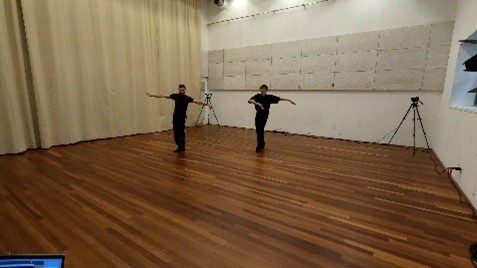
Figure 5: On the top. During the performance: (a) the dancer was smiling, (b) the dancer was laughing, (c) the dancer had a neutral emotion. On the bottom. During the performance: (a) the dancers were laughing, (b) the dancers were smiling, (c) neutral emotion for both dancers.
Video features of the AIST++ and Premiere Dance Motion Datasets.
Video features | AIST++ Dance Motion Dataset | PREMIERE Dance Motion Dataset |
Multi-views synchronised images | 9 cameras | 4 GoPro cameras (see Figure 1). |
Occlusions between dancers or auto-occlusion | 1, 2 or 10 dancers (depending of the sequence). Few occlusion or auto-occlusion. | 1 or 2 dancers (depending of the sequence) with complex occlusion patterns. Specific occlusion features (e.g. see Figure 2). |
Unconventional pose, velocity and acceleration | Floor dance genre: Break, Pop, Lock and Waack, Middle Hip-hop, LA-style Hip-hop, House, Krump, Street Jazz and Ballet Jazz (basic and advanced) | Unconventional pose (e.g. see Figure 3). Motion blur. Contemporary dance/choreography |
Heterogeneous lighting conditions | Constant lighting conditions (white source) with low shading | Strong variations of lighting conditions over time and over areas (e.g. see Figure 4). Strong variations of shading. |
Lack of discriminative features between dancers (except faces) | Few dancers wear dark clothes | Dancers wear dark clothes |
Specific features | In very few videos, dancers express various face emotions. Image size 1920×1080 pixels | In few videos, dancers express various face emotions (e.g. see Figure 5). Image size 5312×2988 pixels |
This dataset will enable us to evaluate the efficiency and robustness of human body pose estimation methods, people trajectory estimation and action parsing methods, against specific features, such as the ones reported in the previous table.
The video content of this dataset, and of archive videos available in the community, will enable us: – to evaluate the limits of the State-Of-the-Art methods; – to compare the efficiency of the best methods in the field of contemporary dance; – to evaluate the potential of archive videos depending of the quality of these videos (in terms of video frame rate, spatial resolution, motion blur, etc.) in regards to the digital creation process.
By Laboratoire Hubert Curien – University Jean Monnet (UJM) team